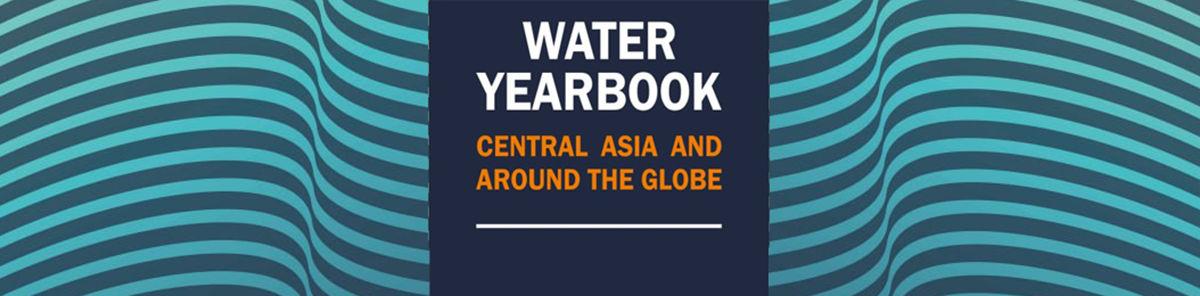
Section 12. Thematic reviews
12.4. Artificial intelligence and water resources management
Prepared by M.S. Valieva, D.R. Ziganshina (SIC ICWC)
The year 2023 will be remembered as a watershed moment in our collective understanding of artificial intelligence (AI). The launch of ChatGPT late last year catalyzed widespread exploration of AI's capabilities, its potential impact (both beneficial and detrimental), and its practical applications in daily life. This phenomenon was reflected in numerous rankings that identified AI development as a defining event of the year, alongside significant geopolitical shifts. Major consulting firms and leading universities conducted extensive research on AI's economic and societal implications. International news outlets extensively covered the technology, highlighting both its promise and its perils.
This review will delve into the specific applications of AI technologies within the critical domain of water resource management, while also examining the potential risks associated with their implementation.
Artificial Intelligence: Key Concepts
Artificial Intelligence (AI) refers to the ability of computer systems to perform creative functions that have traditionally been considered the prerogative of humans. Specific applications of AI include various expert systems, information processing in multiple languages, speech recognition, and systems used for financial trading. Two important concepts that are often mentioned in the context of AI are machine learning and computer vision.
Machine learning is an AI field focused on creating systems that learn and evolve based on the data they receive . One of the best-known machine learning methods is the Artificial Neural Network (ANN) that works by mimicking biological neural networks that exist in a human brain. ANNs learn from training data presented to them in order to capture the functional relationships among the data, even if the underlying relationships are not known or the physical meaning is difficult to explain. This enables the ANNs to discover patterns in data that are often unknown, even to the best experts in the field.
Computer Vision is an AI field related to image and video analysis; it includes a set of methods that empower a computer with the ability to interpret and understand digital images and videos. Examples of computer vision applications include systems for facial recognition, medical diagnostics and driverless cars.
ChatGPT (Generative Pre-trained Transformer) is a chatbot with generative artificial intelligence that can operate in a dialog mode and handle queries in multiple languages. A key feature is its ability to generate code in various programming languages upon request.
Internet of Things (IoT) (IoT) is the concept of a data transmission network between physical objects equipped with built—in tools and technologies to interact with each other or with the external environment.
A short digression
The term "artificial intelligence" (AI) was coined in 1956 at a workshop at Dartmouth College, USA. This workshop focused on developing methods for solving logical, rather than purely computational, problems. Notably, the English term "intelligence" signifies "the ability to reason intelligently," distinct from "intellect." This subtle nuance is often lost in translation, where "artificial intelligence" can carry a slightly anthropomorphic, almost fantastical connotation.
can carry a slightly anthropomorphic, almost fantastical connotation. Shortly after its establishment as a distinct scientific field, AI research bifurcated into two major branches: neurocybernetics and "black box cybernetics." These branches have evolved largely independently, exhibiting significant differences in both methodology and technological approaches.
(Quoted by: T. Gavrilova, V. Khoroshevsky. Knowledge bases of intelligent systems. St. Petersburg: Peter, 2000 - 384 p.)
General trends in AI development in 2023
The latest annual McKinsey Global Survey on the current state of AI confirms the explosive growth of generative AI (gen AI) tools. Less than a year after many of these tools debuted, one-third of respondents reported that their organizations regularly use GenAI in at least one business function. Amid recent advances, AI has risen from a topic relegated to tech employees to a focus of company leaders: nearly a quarter of them report personal use of AI tools for work. Furthermore, 40% of respondents say their organizations will generally increase investments in AI .
According to the BBC. many employees use AI to solve administrative tasks, such as writing simple texts and generating ideas, which helps save time and frees up workers to focus on creative and more complex tasks .
According to forecasts, AI has the potential to both create millions of new jobs and displace many existing ones. The World Economic Forum's Future of Jobs Report 2023 highlights that AI and machine learning specialists, data analysts and scientists, and digital transformation specialists are among the fastest-growing roles. However, AI cannot replace all professions, particularly those requiring common sense, creativity, physical dexterity, and emotional intelligence .
In everyday life, AI has become an integral part, assisting through virtual assistants (Siri, Alexa, Alice), providing recommendations and information on search and entertainment platforms (Netflix, Spotify, Yandex), and more. In healthcare, AI is used for accurate diagnosis and personalized treatment. In digital advertising and financial security, AI enhances ad targeting and fraud detection. In the automotive industry, AI supports the development of autonomous vehicles, potentially increasing safety and efficiency in transportation. With the correct design of requests, AI is also effective in creating educational programs, selecting teaching models, and preparing instructional materials.
Source: World Economic Forum (2023), Everything you need to know about AI in 2023: the 6 must-read blogs
The use of AI in the water sector
The potential applications of AI in the water sector are immense. Below are some of the most compelling aspects of its use in data monitoring and analysis:
• Water Demand Management. AI is capable of uncovering hidden trends in large datasets, enabling utilities to forecast demand, optimize water distribution throughout the day, reduce waste, and meet needs more effectively. Machine learning algorithms can analyze real-time data to adjust water flow and pressure, maintaining a steady supply and minimizing losses during maintenance. AI models trained to consider weather conditions, seasonality, and other factors can identify large-scale issues in water usage and assist in decision-making related to infrastructure, investments, and resources. For instance, Singapore uses intelligent water management systems that use AI to forecast water demand and optimize supply.
• Weather and Climate Forecasting. AI-based water management models, incorporating data from the Internet of Things (IoT), can analyze disparate datasets to assess climate risks and develop adaptive water supply strategies. AI is used to create predictive models that forecast water resource availability based on climate change scenarios. These models can integrate a wide range of data, including historical weather patterns, current climate trends, and future climate projections. By analyzing this data, AI can provide valuable insights into the impact of climate change on future water availability. A study by Stanford University demonstrated AI's potential in forecasting groundwater recharge, with the AI model achieving high accuracy in predicting groundwater replenishment rates .
Researchers from Nvidia and Google have embarked on developing large AI models, known as foundation models, for weather forecasting. These models are capable of providing more accurate forecasts compared to existing numerical models and have lower computation costs . Some of these models can predict weather conditions beyond seven days, opening new avenues for scientists . Methods for assessing precipitation intensity using video streams from smartphones or surveillance cameras, as well as unconventional IoT data sources, are advancing. Technological advances in image processing and computer vision enable extraction of diverse features, including identification of rain streaks enabling estimation of the instantaneous rainfall intensity (Allamano et al, 2015). Recent AI and machine learning approaches rely on the use of autoencoders, deep learning and convolutional neural networks to address the problems. Companies such as WaterView (Italy), Hydroinformatics Institute (Singapore), as well as universities (Southern University of Science and Technology China, Shenzhen) have proposed and implemented practical approaches to weather hazards in energy, automotive and smart cities application domains (Jiant et al, 2019) .
• Forecasting and Mitigating Potential Water-Related Risks. Flooding poses a persistent threat in many urban areas and communities. AI can be used to analyze weather and water level data, as well as to predict the likelihood of flooding. This capability enables local authorities to implement preventive measures and evacuate individuals from high-risk areas. IBM employs AI to develop predictive analytics tools and optimize water resource management. Their solutions assist cities and industrial enterprises in effectively managing water resources.
The Serbian company Vodena is developing an innovative solution, VodostAI, to combat flooding in the Western Balkans, where damages amounted to €300 million in 2023 and over one million people were affected. The VodostAI platform employs AI and the Internet of Things (IoT) for continuous monitoring and updating of models based on new data. Vodena automates data collection using intelligent sensors and machine learning algorithms, enabling accurate water level forecasting and timely notifications .
In 2018, Google launched an AI-based flood forecasting system in India, a country frequently affected by severe flooding. The system employs a combination of machine learning, hydrological models, and the most up-to-date weather data to predict areas at risk of flooding. According to a report by The Times of India, the system successfully provides timely and accurate flood threat alerts, allowing local communities to take necessary precautions and potentially saving countless lives .
Interactive web map
Source: Sakti Prajna Mahardhika and Okkie Putriani (2023), IOP Conf. Ser.: Earth Environ. Sci. 1195 012056
• Optimization of Water Consumption. This approach is based on the concept of automated data analysis. The platform developed by Plutoshift, which employs AI, collects data from various sources and then processes it using machine learning algorithms to provide real-time insights for optimizing water use. The platform can identify patterns and trends that are difficult for humans to discern and forecast future water consumption based on historical data and current conditions. By optimizing water use, businesses can significantly reduce their operational costs. For instance, a beverage company utilizing Plutoshift’s platform was able to save $140,000 annually .
• Optimization of Irrigation Systems. AI-driven irrigation systems optimize water resource use by utilizing data on weather conditions, soil moisture levels, and crop requirements. These systems ensure that agricultural crops receive the precise amount of water needed at the appropriate times, reducing waste and improving yield. An example of such technology is the AI-based irrigation systems from Netafim. Economic benefits of intelligent irrigation systems include substantial cost savings through reduced water and energy consumption. Although the initial investment in these systems may be significant, they typically pay off within one to three years through water savings, reduced labor costs, and increased yields. Additionally, improvements in soil fertility and environmental sustainability provide long-term advantages .
• Enhancing Water Supply Efficiency. As highlighted in the Water Technology Trends 2023, AI models can be employed to optimize water supply systems, minimize costs, reduce water losses, and improve the energy efficiency of infrastructure. This can contribute to lowering operational and maintenance expenses while enhancing access to clean water.
The AI-based WaterScope solution detects leaks in municipal water supply systems and provides real-time alerts to prevent water loss. Siemens utilizes AI and the Internet of Things to deliver digital solutions that enhance water resource management and operational efficiency. The company Fracta has leveraged AI capabilities to refine methods for detecting and addressing losses in water infrastructure. Fracta employs machine learning to predict the likelihood of pipe failures in water supply systems. The AI system processes extensive datasets, including pipe material, age, diameter, and historical leak data. Machine learning algorithms are then applied to these data to predict where leaks are most likely to occur. This leak prediction method has been practically implemented with excellent results. For instance, Fracta's AI-based leak detection system was adopted by the Murfreesboro Water Resources Department in Tennessee, USA, and identified potential leaks with up to 69% accuracy, significantly surpassing industry standards. By forecasting likely leak locations, the system enables proactive maintenance, thereby preventing costly and damaging pipeline failures .
• Detection of Crop Growth Anomalies. This process involves identifying irrigation issues and implementing timely corrective actions. When planning crops, AI analyzes data related to crop selection parameters, enabling optimal crop choices and the development of predictive models for future water supply needs. This supports the creation of resilient and sustainable agricultural systems that are adaptable to changing water availability conditions.
• Water Quality Monitoring. The Netherlands, renowned for its innovative water management strategies, is at the forefront of integrating AI into water quality monitoring. A notable project in this regard is the "AI for Water Quality" (AI4WQ) initiative, undertaken collaboratively by water authorities, research institutions, and technology companies. The AI4WQ project utilizes AI algorithms to process vast datasets collected from various sensors installed in water bodies throughout the Netherlands. These sensors measure real-time data on temperature, pH, turbidity, and concentrations of various chemical and biological substances, identifying patterns and trends that may indicate changes in water quality .
The U.S. Environmental Protection Agency (EPA) also employs AI for real-time water quality monitoring and the detection of pollutants. Hitachi leverages AI to provide advanced water resource management solutions, including predictive maintenance and optimization of water distribution networks.
Real-time monitoring and forecasting of water quality
Source: Hesam Kamyab, Tayebeh Khademi, Shreeshivadasan Chelliapan, Morteza Saberi Kamarposhti,
Shahabaldin Rezania, Mohammad Yusuf, Mohammad Farajnezhad, Mohamed Abbasi, Byong Hun Jeon,
Yongtae Ahn (2023). The latest innovative avenues for the utilization of artificial Intelligence
and big data analytics in water resource management. Results in Engineering 20 (2023) 101566
Peter Ma from Intel has developed a prototype system that uses AI methods for detecting bacteria in water. This system features a digital microscope connected to a portable computer running Ubuntu and an Intel Movidius neural computer, enabling autonomous analysis and real-time mapping of contamination zones.
The platform, based on Intel Xeon processors, is designed for deep learning and computational tasks. Peter utilized Intel AI DevCloud to train the AI model and the Intel Movidius Neural Compute Stick for real-time water testing.
The entire testing system is composed of readily available components, such as a digital microscope and inexpensive computing devices, with a total cost not exceeding $500. The underlying convolutional neural network enables the identification of current bacteria, such as E. coli and Vibrio cholerae, with the capability to extend the detection range .
Базовая тестовая система
Source: Datafloq (2018), AI-Driven Test System Detects Bacteria in Water
AI also enables the detection of unregistered carcinogenic contaminants globally. Chinese scientists have developed a new platform for the detection and precise identification of unknown PFAS (per- and polyfluoroalkyl substances) in the environment. The platform employs an advanced molecular screening tool integrated with machine learning, allowing for the identification of 733 PFAS in wastewater, of which 17 groups were previously unknown. Additionally, 126 PFAS were detected in samples from 20 countries, including 37 new and 81 previously unrecognized substances. The platform achieves an accuracy of 58.3% with a low false positive rate of 0.7%, significantly surpassing other methods. This advancement provides opportunities for better risk management and enhances the study of synthetic chemicals' impact on health and the environment .
• Air Quality Monitoring. AI can be utilized to monitor air quality at water treatment facilities. This capability can detect and prevent air pollution that may adversely affect water quality.
In addition to fundamental research and development, it is crucial to focus on the practical application of innovations, including prototype development, localization, and user engagement. In this context, the advancement of AI technologies contributes to addressing the following challenges:
• Organizing Unstructured Earth Data and Localizing Models. Earth sciences rely on vast amounts of unstructured and disorganized data, with over 100 terabytes of satellite imagery collected daily. Recent research and developments illustrate AI's ability to optimize these data. For example, Google Earth Engine, a leading platform for Earth observation, integrates various satellite images and geospatial data with analytical capabilities. AI also advances Earth sciences by adapting global models to local conditions through transfer learning . This method leverages previously studied information about specific areas to address tasks such as predicting forest fires in particular locations. This is especially crucial in data-sparse regions, as it helps bridge information gaps and effectively utilize extensive observational data.
• Simplifying Model Understanding. Generative AI, based on large language models (LLM), facilitates interactive engagement with data users and simplifies the understanding of complex processes. By providing a GPT-like interface, it enables users of all skill levels to interact with climate and hydrological data tailored to their needs.
• Accelerating the Prototyping Stage in Technology Development. AI can shorten the deep tech cycle, particularly in prototyping, speeding up the introduction of essential technologies. In materials science, AI accelerates discovery and design, crucial for climate mitigation (such as improving lithium-ion batteries and solar cells) and adaptation (developing fire-resistant materials). Traditional methods, which calculate material properties from scratch, consume significant time, costs and computational resources. Up to one-third of global supercomputing power is used for materials science. AI now predicts new material properties without exhaustive initial calculations. It does this by learning relationships between atomic structures and their properties, and suggesting optimal configurations. While this is a nascent space with limitations (e.g. documented “hallucinations” in discovery processes), there is significant potential for innovation. For example, GenAI is tackling the inverse design problem, which starts with a desired property (e.g. resilience to extreme weather) and reverse-engineers its design .
Risks of AI Deployment
Currently, it is challenging to definitively assess the consequences of using AI, as people tend to overestimate the short-term impacts of new technologies while significantly underestimating their long-term effects. Overall, experts highlight the following risks associated with the deployment of AI, including in the water sector:
• Data Volume. Effective AI models require large volumes of high-quality data, which may not always be available, particularly in developing countries.
• Limitation. AI models are trained on limited data, which can lead to mediocre or insufficient results. This limitation may hinder innovation and development, as excessive reliance on AI without critical analysis can slow progress . The ease of adopting new technologies may distract from addressing the core needs of clients and employees. It is crucial to critically evaluate AI outcomes and avoid a "set and forget" approach, which can result in a loss of expertise and critical thinking . AI-generated forecasts are not infallible and should be used in conjunction with other forms of analysis and expert evaluations.
• Responsibility. Daniel Kiley from HWL Ebsworth Lawyers emphasizes the importance of maintaining accountability when using AI. Organizations must ensure the proper functionality of AI tools and take responsibility for their outcomes. The use of AI should be justified, and it is crucial to avoid shifting blame onto the tool if issues arise.
• Development costs. The costs associated with developing AI software can vary widely depending on numerous factors. Key factors include data costs, project complexity, infrastructure, development, deployment, regulatory compliance, and ongoing maintenance. On average, the costs for AI software development range from $50,000 to $500,000 for small to medium-sized projects and from $500,000 to $5 million for large-scale projects. While these costs can be substantial, the potential benefits of implementing AI—such as increased efficiency, reduced expenses, improved customer service, innovation, and optimized data use—often justify the investment.
• High Power Consumption. The International Energy Agency (IEA) anticipates that the advancement of AI technologies will double the demand for electricity . Data centers, numbering over 8,000 globally (with 16% located in Europe), consume substantial amounts of energy for both server operations and cooling. According to 2023 estimates by the IEA, data centers account for 1-1.5% of global electricity consumption. Their CO₂ emissions are approximately 1% of the global total, comparable to the aviation sector . From 2024 to 2026, electricity consumption could potentially double, reaching the total electricity consumption level of Japan. In 2023, the share of electricity used by data centers increased to 20%, up from 18% in 2015. Queries to AI chatbots may consume up to ten times more energy than traditional Google searches, while generative AI systems can use up to 33 times more energy than conventional software. This rising energy consumption contributes to increased CO₂ emissions, as it is predominantly powered by fossil fuels.
• Water Consumption. Data centers use a significant amount of water for cooling purposes. For instance, in the United States in 2021, approximately 7,100 liters of water were used per megawatt-hour of energy. Google’s data centers alone consumed 12.7 billion liters of fresh water. This issue becomes particularly critical in water-scarce regions, especially in the context of global warming and extreme temperatures .
• The use of AI also raises ethical and privacy issues related to data collection and usage, necessitating transparency and accountability. Ensuring that data practices are ethical and that privacy is protected is crucial in the deployment of AI technologies .
Source: TechMagic (2024), AI Development Cost: Analyzing Expenses and Returns
Legal Regulation of AI
The rapid development of AI technologies and the associated risks necessitate appropriate legal regulation. Many countries are in the process of establishing legal frameworks for AI implementation, but a well-established and cohesive structure is not yet in place. The European Union and China are currently leading efforts in this area.
On December 8, 2023, negotiators from the European Parliament and the Council reached a preliminary agreement on the AI Act, aimed at ensuring protection against high-risk AI applications for fundamental human rights, democracy, the rule of law, and environmental sustainability, while also promoting innovation. Specifically, the agreement includes provisions on the overarching objectives of AI; restrictions on the use of biometric identification systems by law enforcement; bans on social scoring and using AI to manipulate or exploit user vulnerabilities; the right for consumers to file complaints and receive substantive explanations; and fines ranging from €35 million (or 7% of global turnover) to €7.5 million (or 1.5% of global turnover) . The agreed text now needs to be officially adopted by the Parliament and the Council. The proposed legislation has sparked mixed reactions: an open letter signed by over 150 European business leaders (from Renault to Heineken) highlights concerns that the law may negatively impact businesses and threaten regional competitiveness, while failing to address the very issues it was designed to resolve .
In China, specific regulatory measures concerning AI have already been implemented, but these address a narrow range of issues. A comprehensive law establishing general rules for the AI industry is still lacking. In August 2023, a preliminary draft law was prepared by a group of researchers from the Chinese Academy of Social Sciences and published for discussion as the Model Law on Artificial Intelligence, Version 1.0 (Expert Draft Proposal). Notable features of the Chinese approach include its iterative nature, allowing for adjustments with each new step, and its sector-specific focus .
In March 2024, the United Nations General Assembly adopted a resolution titled "Seizing the opportunities of safe, secure, and trustworthy artificial intelligence systems for sustainable development". Although non-binding, this resolution represents the first UN-level document aimed to establish a framework for the development and regulation of AI technologies globally. The document begins by reaffirming commitment to international law, particularly the UN Charter and the Universal Declaration of Human Rights. The reference to human rights is particularly pertinent regarding the ethical and secure use of data. The resolution calls for bridging the digital divide in AI and other technologies, both between and within countries, to achieve the 17 Sustainable Development Goals (SDGs) .
The resolution outlines measures for ensuring data privacy, advocating for the safe development of AI, especially when dealing with sensitive personal information such as medical, biometric, or financial data. Member states and relevant stakeholders are encouraged to monitor AI systems for risks and assess their impact on data security and privacy protections throughout the entire lifecycle of these systems (6.e, p5/8). The repeated references to "the entire lifecycle" imply a comprehensive regulatory approach to AI, commencing with the "training" phase, where attention to data privacy in selection and usage is critical, and extending through technological development to consumer delivery. Impact assessments on privacy and detailed product testing during the development process are proposed as mechanisms for data protection and safeguarding fundamental rights to privacy . The document also affirms that states should execute their responsibilities in accordance with national legislation, thus providing substantial latitude for states in implementation.
Conclusion
In the context of global climate change, population growth, and increasing water scarcity, AI emerges as a crucial tool for ensuring a sustainable future in water resource management. The use of AI not only aids in effectively addressing existing water supply challenges but also opens up new opportunities for achieving water security and sustainable development. For instance, smart irrigation systems can significantly enhance water distribution efficiency in agriculture, while leak detection systems can help reduce water losses.
At the same time, integrating AI into water resource management presents several challenges that must be addressed. Firstly, the need for high-quality data for training and optimizing AI models can be hindered by a lack of or incomplete data availability. Secondly, the implementation of AI technologies involves significant costs, which can be a barrier to widespread adoption, particularly in developing countries or regions with limited resources. Thirdly, issues of ethics and data privacy arise, necessitating careful regulation and adherence to standards to prevent misuse and protect user rights.
Therefore, the rational application of digital technologies should be coupled with adherence to ethical standards, effective data and resource management, and continuous monitoring and adjustment of methods. It is crucial that the integration of AI into water resource management is approached with awareness and balance, focusing on maximizing its potential benefits while minimizing possible risks and negative consequences.